Data analytics is the process of collecting and organising data to identify trends and patterns in order to make predictions and improve decision-making. It is crucial for tackling specific issues or challenges in an organisation as it can provide insight into customer behaviour, competitors’ patterns, and so much more.
In this article, we’ll explore what data analytics is, its key concepts, the different types and techniques, tools for analysing data, and more. Let’s get started!
Quick Summary
- Data analytics involves collecting and organising data to make predictions and guide decision-making.
- Data analytics is very important for tackling specific issues and challenges in an organisation.
- There are various techniques and tools for data analytics, such as data visualisation using tools like dashboarding, data mining, and spreadsheets.
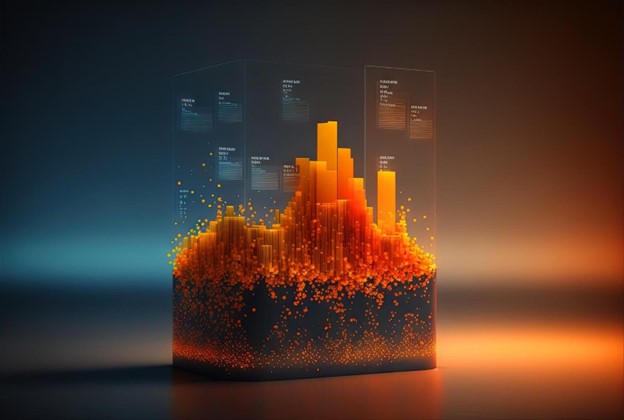
What Is Data Analytics?
Data analytics is a multifaceted concept that goes beyond mere data analysis to include a broad range of techniques used for extracting insights from data. From theories of data collection to the practical application of those theories, data analytics has become invaluable for improving problem-solving and decision-making.
With data analytics, a business or organisation can discover trends and patterns that would have been missed through mere observation. For example, a tech company might look at consumers’ phone usage patterns to figure out their next app to design and what features would make it outstanding. Similarly, a fashion store may record sales patterns and other social indicators and then analyse the data to better curate its collection and set prices.
Data analytics is often mixed up with data analysis. Although they sound alike and are related, they have distinct meanings. Data analysis is a component of the data analytics process. While data analysis focuses on extracting meaning from sets of data, data analytics refers to the entire spectrum of collecting, processing, analysing, and interpreting data to drive strategic decisions.
Steps Involved In Data Analytics
Data analytics involves a series of strategic steps that translate raw data into actionable insights for informed decision-making. Each step in this process is interconnected, and skipping any step can impact the effectiveness of data-driven decisions. Let’s take a look at them
1. Identifying Business Objectives
The first step in data analytics is to clearly define the goals. This involves understanding the specific business challenges or opportunities and determining how data can help in addressing these.
2. Data Collection and Integration
This step involves gathering data from various sources, which could include internal systems, online channels, or third-party data providers. After data is collected, it is then integrated into a unified format, which is vital for effective analysis.
3. Data Cleaning and Preparation
This is typically the most time-consuming step, and it involves preparing the data for analysis. Data cleaning includes removing inaccuracies, handling missing values, and standardising data formats.
4. Data Analysis
Here, the cleaned data is subjected to various analytical techniques. This can range from basic descriptive analytics that summarises past data to more complex predictive or prescriptive analytics that forecast future trends or prescribe solutions.
5. Data Interpretation and Insight Generation
This stage transforms complex data findings into understandable insights. Identifying trends, patterns, and correlations enables organisations to gain a deeper understanding of their operations, market, and customers.
6. Decision Making and Strategy Development
Armed with insights, organisations can then make informed decisions. This step involves applying the knowledge gained from data analytics to formulate strategies, make policy decisions, or drive operational improvements. The goal is to use the insights gleaned from the data to create tangible business value.
7. Implementation
This step involves putting the decisions or strategies into action. Whether it’s launching a new product, adjusting marketing strategies, or changing operational procedures, implementation is where the theoretical benefits of data analytics are translated into practical outcomes.
8. Monitoring and Evaluation
The final step is to continuously monitor the results of the implemented strategies and evaluate their effectiveness. This ongoing process helps in refining strategies, adjusting objectives, and further optimising the data for future decision-making.
What Are The Types of Data Analytics?
There are four main types of data analytics that are often used in data science. These are:
Descriptive Analytics
This type of analytics is used to describe occurrences over a period of time. It is one of the most commonly used types of analytics that focuses on past events. It is easy to use because the data is already available for reports and action. This is very similar to how Google Analytics works.
Descriptive analytics help companies and businesses gain insight into certain questions, such as how much sales were recorded over the past month, the number of new customers, and what product customers focus on more. Basically, this type of analytics helps data analysts answer the question, “What happened?”
Diagnostic Analytics
This is similar to descriptive analytics, but instead of providing insight into what is happening, diagnostic analytics addresses why something occurred within the data collected. This requires various data inputs and is more beneficial for diverse cases than machine learning or predictive analytics.
Diagnostic analytics can help businesses or organisations answer important questions such as, why did customers unsubscribe from our newsletter? Why did more iOS users uninstall our app last month? Why are fewer people walking into physical stores? And so on.
Predictive Analytics
This is more advanced than the other types of analytics we mentioned earlier. It uses machine learning to provide insight into events likely to happen in the near future based on historical data. This data comprises information from descriptive and diagnostic analytics used to build predictive analytic models.
Predictive analytics is helpful in addressing future outcomes, such as determining when customers tend to use a service more, predicting the need for routine maintenance, and avoiding customer dissatisfaction.
Prescriptive Analytics
This type of analytics is used when you need to take a course of action. It is a really guided form of analytics that suggests, prescribes or guides you towards the best possible action. Prescriptive analytics is basically an incorporation of other types of data analytics, including marketing automation, for effective decision-making.
This is more complex than other types of analytics, which is why it is usually used in highly specialised businesses or organisations. Prescriptive analytics is useful for automatically adjusting existing systems based on anticipated outcomes and external factors and implementing additional safeguards based on incident reports.
Different Techniques of Data Analytics
Other than the types of data analytics, there are also different techniques used to process data for meaningful insight. Some of the most popular ones include:
Regression Analysis
This is the analysis of the relationship between a set of variables to determine how one or more variables may affect the dependent variable in order to establish patterns and trends. This is a great way to make predictions about future trends.
Factor Analysis
This technique is used to shrink a large number of variables or data sets into a smaller set of factors. The aim is to identify underlying trends that connect multiple observable variables. Factor analysis enables you to explore concepts that are not easy to see, such as fitness, satisfaction, and wealth.
Cohort Analysis
Cohort analysis is used to group data sets based on similar characteristics. This makes it easier for data analysts to identify trends and patterns.
Cluster Analytics
This technique aims to identify structures in a dataset. The objective of cluster analysis is to categorize different data points into groups based on their similarities, while ensuring these groups are distinct from each other. Clustering is applied to understand the distribution of data within a dataset.
Time Series Analysis
This is used to track and identify trends over a sequence of data points, which evaluates the same variable over time. Time series analysis is used to single out recurring trends or assist financial projections.
Tools For Data Analytics
Data analysts have always relied on various technological tools and statistical approaches for data mining and interpretation. Some of the best tools these days include:
- Python
- R
- Looker Studio
- Microsoft Power BI
- SAS
- Jupyter Notebook
- Apache Spark
- Tableau
Each of these tools provides powerful resources for extracting meaningful insights from complex data sets. They allow data analysts to effectively tackle a wide range of analytical challenges, adapting to various data types and business needs.
Embracing the Power of Data Analytics for Smarter Decision-Making
So, there you have it! All you need to know about data analytics. There are many aspects to it, but when used properly, data analytics can help individuals and organisations scale their businesses better. Today, the world is more reliant on data and statistics than it was several decades ago.
Data analytics may seem daunting, but the good news is that you don’t need to handle it all by yourself. If you need help, you can reach out to a reliable data marketing agency and get the results you want.